GovLoop’s online training addressed the concept of “bad data” with a panel of experts:
- Bobby Caudill, Global Industry Marketing, U.S. Public Sector, Informatica
- Murthy Mathiprakasam, Principal Product Marketing Manager, Informatica
- Lori Walsh, Chief, Center for Risk and Quantitative Analysis, U.S. Security and Exchange Commission
Titled “Beware Bad Data”, this webinar highlighted how modern technology and tools are able to help government agencies transform their bad data into the knowledge essential to informing policy.
First off, what is bad data?
Walsh broadly defined bad data as, “any data that impedes your ability to analyze and draw insights.” When an agency is using data for analytical purposes or to inform policy, it is necessary to remove the “badness.” However, Walsh pointed out that badness runs on a spectrum. Data could be bad for one project but perfect for a another. Therefore, it is essential to truly identify what you need the data for.
Mathiprakasam added that when people try to transform bad data into usable data by hand, they often run into further challenges while wasting time. Technology can reduce the time necessary to clean bad data and get you the results needed.
What are the causes of bad data?
The causes of bad data come in many forms. Walsh identified a few: inconsistent names or fields, misleading or inaccurate data, misinterpretation, and more. Most significant for Walsh was the custody risks that come with utilizing spreadsheets. When someone handles data that you had used a year ago, that person has the ability to alter any of it. Consequently, the data analyses that you ran a year ago may not be possible now because of any alterations that may have occurred since.
Utilizing bad data can have significant impacts on your ability to get any useful insights from the data. For example, more time can be added to your process, which wastes agencies resources. What’s more, inaccurate or misinterpretation of the results can lead to ill-informed policies that negatively impacts public service. Finally, using bad data creates a vicious cycle as new bad data is produced.
Now that we know what bad data is, how do you avoid using it?
Bottom up approach
Walsh is on the business side and relies on her IT counterparts to provide her with appropriate analyses. Because she is getting the data from someone else, she needs to learn the data inside and out. This begins with starting from the bottom and figuring out the exact source of the data.
From there Walsh said she must understand how she wants to use the data. She talks to experts to create a joint question that they want answered. Next, she identifies the correct tools that will help her extract the correct insights from the analyses while removing any “badness.”
Additionally, Walsh and her team have begun using visualization tools to perform more robust, deeper searches through the data to extract the desired information. This allows them to bypass cleaning steps and save more time.
Top down approach
Mathiprakasam offered a slightly different approach that seeks to first understand the ultimate social need. For example, if an agency wants to identify specific health services needed in an area, what data do they need for this? And who is accountable for the completion of that social mission?
To answer these questions, collaboration with policy makers on the search for appropriate data sets is necessary. Additionally, it is important to consistently monitor the evolution of the mission so that the data can be best applied throughout the process.
Lastly, the data analysis is run to find the results needed to inform the policy.
Tools at every level
One of the audience members was interested in what tools Walsh and Mathiprakasam utilize to secure good data. It became clear that there are countless tools that can be applied at every level of the data analysis process.
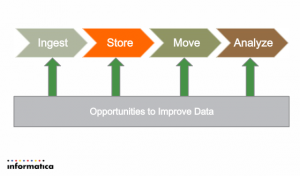
- Ingestion: When you need to bring data in quickly, Informatica offers tools that can provide near real-time transfer of data into an analysis
- Storage/Processing: Several different tools can be used while fixing and altering data. Walsh and her team are fond of SaaS to improve data and get more comparable fields. Informatica also has different types of transformation techniques to develop alternate data visuals for each unique need.
- Moving/Securing Data: Informatica offers several tools for data protection. For example, you can mask data so only certain people in the agency have access to the raw information. Or you can use a tool that allows you to see specific people that have looked at or altered the data.
- Analysis: Walsh is a big fan of Excel spreadsheets. Functions in Excel allow her to create a repeatable process across data sets. Therefore, results are more consistent for anyone that runs the analysis.
Moving forward with good data
Mathiprakasam concluded that the hardest part of dealing with bad data is that each case is different and sometimes data sets require custom approaches. Moving forward with good data often requires a technical approach to enterprise data management (EDM).
EDM is a “set of processes that are discretely definable, repeatable and read to be automated,” according to Bobby Caudill. Technology can help solve problems through extraction and standardization of complex data that might have a level of “badness.”
Technology and solutions are constantly overlapping with data analysis. However, with the correct tools and knowledge, bad data can convert to meaningful results and ultimately inform positive public policy.
Want to know more? View to the entire online training on-demand!
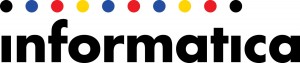
Really enjoyed this presentation was informative, practical, use full.
Thanks
[…] To learn more about how you can turn bad data good, listen to the whole online training on-demand! Also, check out the complete training recap! […]