As government agencies advance in their digital transformation journey and consider how to implement technology to improve service delivery, more attention is being given to how data can be used to solve challenges around digital services.
Data itself is not an issue – government agencies have access to plenty of data. It’s how the data is influenced, gathered and managed that has to be analyzed before it can be used in the development and adoption of new tools – especially AI-powered technology.
Artificial intelligence (AI) and machine learning (ML) are powerful tools that can optimize a modern data system; however, they can’t be implemented instantly with positive results. These tools rely on systems and a culture that have been intentionally designed to work together. We believe that addressing the workforce “platform” and the digital “platform” together can truly achieve a continuous improving, innovation culture based on learning.
According to the Harvard Business Review, “The biggest obstacles to creating data-based businesses aren’t technical; they’re cultural. It is simple enough to describe how to inject data into a decision-making process. It is far harder to make this normal, even automatic, for employees — a shift in mindset that presents a daunting challenge.”
In a data-driven culture, leaders push to make sure needs are addressed across the board and utilize their tools, processes, and technology to reduce the burden on individuals. Below is a useful maturity model for data-driven cultures that can function as a map and enable conversations and decisions about how powerful tools can be implemented in a way that increases organizational and individual capacity.
We recommend using two maturity models to assess data-driven culture evolution: data maturity and analytics maturity.
What is data maturity?
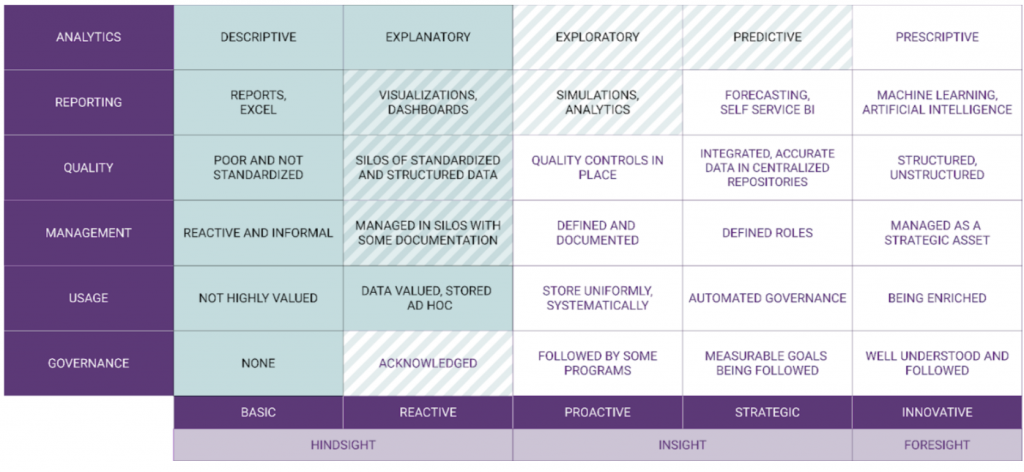
- Basic. The organization recognizes the importance of collecting data, but still needs to build the structure to do serious analysis.
- Reactive. Data is starting to be collected into a single data warehouse to enable a holistic view.
- Proactive. The data strategy is mature enough to enable the building of statistical models.
- Strategic. Data is being used to make predictive models as a way to make decisions about the future.
- Innovative. Machine Learning (ML), Artificial Intelligence (AI), and Robotic Process Automation (RPA) are being used to automate decisions, and data is being enriched with third-party information.
What is analytics maturity?
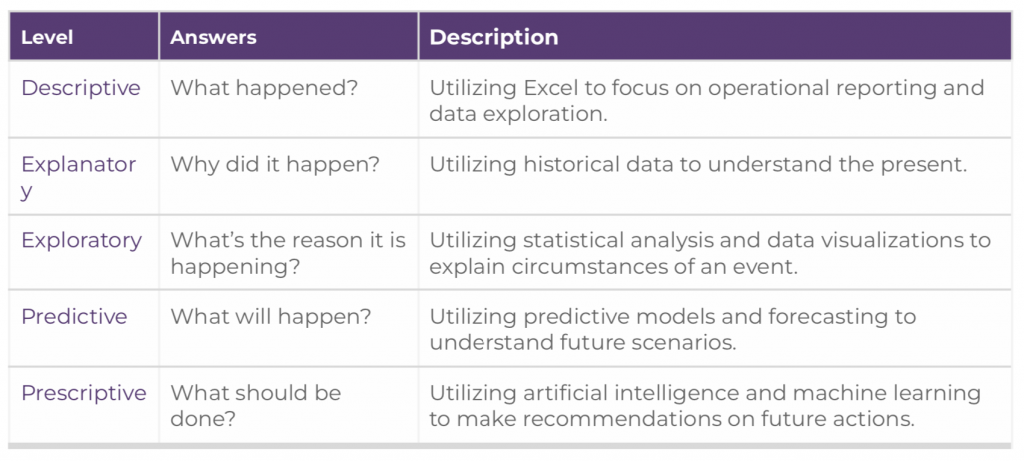
A data-driven maturity model
Before we can evolve into a data-driven culture, we have to understand where we are in our usage and analysis of data. Utilizing maturity models to understand the process and path of change is beneficial for organizations because they can delineate clear and attainable metrics for success. They also can empower leaders to make informed decisions about areas to prioritize at a given time and to identify objectives that resonate with specific needs.
A data-driven culture is critical to the successful adoption and trusted implementation of AI-powered technology to improve and streamline the digital service experience.
Greg Godbout, an entrepreneur and experienced solution architect, is the Chief Growth Officer for Fearless, a full stack digital services firm based in Baltimore.
Leave a Reply
You must be logged in to post a comment.