DeepSeek, Chat GPT, LLMs, hallucinations, machine learning, AI, chat bots, social media tracking, TikTok data mining, Facebook’s targeted ads, phones listening to us … talk about data permeates every bit of our lives, so it is understandable that we might not understand the particulars of how all of those technologies work — let alone the intersection of them.
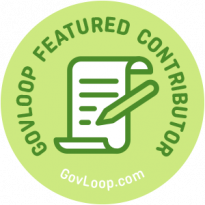
Data. Data is where they all intersect, and it’s the fuel that all apps run on. You don’t have to be a data scientist to understand the basics about data, but data literacy is a must have for the future.
Data Literacy Is More Than Pie Charts and Graphs
Merriam-Webster defines being literate as being able to read and write. But literacy is about more than the ability to read — it’s about the ability to understand and make decisions based on what you read. Think about health literacy, where if you don’t understand the implications of the information providers give you, your ability to make those decisions may be given to someone else. For example, when a loved one of mine had a head injury, medical staff wouldn’t let them consent to procedures, even though they were talking cogently — I had to do it. This is how important it is to know enough to be able to make sound decisions, not just enough to understand the words in a sentence.
Data Literacy Includes Understanding Data Quality
Wikipedia defines data literacy as “the ability to read, understand, create, and communicate data as information.” Some definitions, like this one from Tableau, are simple, but light on details. Gartner’s definition is straightforward and emphasizes how important context is to data literacy — much like understanding details about health issues is critical to making health decisions. Unesco’s attempt references communication and understanding. Some definitions emphasize that data literacy involves evaluating data to make data-driven decisions.
All of these definitions are correct, but they lack an acknowledgement of how important data quality is to comprehension. You must have good data to base decisions on, and you must be able to identify good and bad data. As IBM puts it, “Data quality measures how well a dataset meets criteria for accuracy, completeness, validity, consistency, uniqueness, timeliness and fitness for purpose.”
Since I think understanding data quality is essential to data literacy, I define data literacy as the ability to:
- recognize good (and bad) data
- understand how data relates to other data
- connect how data relates to your specific field or domain, and
- be able to interpret data in a way that lets you understand something that you need to know
In addition, I think data literacy lies on a continuum — think of the difference between being able to read and write well enough to get an A on a college essay, and writing a novel. There’s competency at one end, and there’s fluency at the other.
Government leaders should be able to parse information from their experts and make decisions based on it. I’d equate this with being able to write a solid college essay. The folks providing the data with which to make decisions likely are technologists and should be fluent in data.
All points on the spectrum should understand why data is important, the general principles around what data can do, what makes good data, and how it drives productivity and functionality.
Here’s a handy comparison chart.

Why Data Literacy Is Important
Data is important to us only because there is something that it tells us that we need to know. To paraphrase Professor Richard W. Hamming’s famous quote, “The purpose of [data] is insight, not numbers.” Data literacy moves us beyond Excel sheets and PowerPoint graphs to learning something meaningful.
My next few blog posts will explore the data literate organization, starting with exploring data quality. Please reach out with questions or observations.
Evelyn L. Kent is the owner of Bacon Tree Consulting, a firm that helps clients with digital transformation; systems analysis; validating AI and machine learning models; data forensics; metadata management; text analytics; and building semantic models, ontologies, and knowledge graphs. Evelyn helps organizations find and solve the data barriers that block enterprises from innovation, new markets, and efficient operations. She regularly writes and presents on all things data.
Leave a Reply
You must be logged in to post a comment.