Artificial intelligence (AI) often works by magic. While seemingly nobody knows how it operates, people are amazed and inspired by the successes that AI has achieved.
Or rather, when AI operates functions smoothly, people are amazed and inspired. But when it doesn’t, people are left frustrated and perplexed.
The problem with AI is that the science behind it remains arcane to most, and even the smartest AI minds can have difficulty predicting the successes and failures of projects from start to end. AI often is a “black box,” consisting of multiple components but producing results that can be difficult to track and account for – leaving it understood by few and difficult to correct.
“To be totally clear, we’re still in the infancy of this technology in some ways,” Chris Sexsmith, Cloud and AI/Machine Learning (ML) Field Strategy Lead for Public Sector at Red Hat, said during GovLoop’s virtual summit on Wednesday.
The early stages of AI mean risks abound in its usage. While there have been successful rollouts, such as with Tesla and Amazon Echos, the public still perceives AI with as much anxiety as anticipation. And that’s fair, especially in government offices that need to be risk-averse to protect constituents.
Derailed artificial intelligence projects can collapse in difficult circumstances or – possibly worse – make decisions based on bias and produce inaccurate or unfair results. These are legitimate worries, Sexsmith said, because AI and ML are driven by data as much as coding. Whereas employees can trace other emerging technologies’ failures back to manmade flaws in the coding, problems with AI can come from undetected skews in datasets that train and inform models.
And while codes are reviewable, often the information that AI processes is sensitive and thus cannot be analyzed by external sources.
“How do I train a model and make that model actually usable to another team, without giving them access to the data, because there are obviously privacy concerns with some of that data?” Sexsmith said.
Viewers of the online training, which centered on how agencies could overcome common barriers to AI, gave numerous reasons as to the limited adoption of AI. Just about one-tenth of viewers knew their agency was using AI, while more than three times as many knew theirs wasn’t. Most were unsure.
Governments are being pushed more and more so to AI, however. As the landscape of foreign states and private sector companies decide to take the plunge into artificial intelligence technology, the onus is on U.S. governments to respond. Some that are in direct competition with other actors, such as the Defense Department, already have.
For those who haven’t, however, AI adoption is becoming more tenable because of open source methodology. Open data that spans every use case can be shared across departments and sectors securely, and hosts can support models and pilots for AI technology that is based off of this data.
Open data is subject to the eyes and analyses of many, meaning that biases and flaws in the sets are likely to be caught and corrected, as opposed to strictly internal data. Furthermore, these expansive systems can allow for more comprehensive and accurate modeling, as the instances for AI expand.
“Data is just as important, if not more important than the code,” Sexsmith said.
The development of quantum computing and edge devices will expand the processing and depth of these datasets. Therefore, governments need the right systems and processes to control their data and AI projects. Open data allows agencies to operate transparently and collaboratively to deploy reliable AI.
“Turning it into a black box and not having control or insight of what you’re into, that’s likely to cause you problems as policy is developed,” Sexsmith said.
Red Hat and open source methodologies are well positioned to allow agencies to steer the future of their AI projects. Red Hat has launched an open data hub, a data and AI platform designed for hybrid cloud, that allows for the integration of open source AI projects. Industry partners can support the step-by-step needs of data collection, modeling, experimentation and deployment.
Sexsmith encouraged agencies to operate in a pattern to own and fine-tune their data projects.
In rolling out AI and ML projects, collect and visualize data, and analyze it to discover patterns, Sexsmith said. From there, model and optimize the product, and incorporate DevOps during deployment to monitor and optimize the results.
“We’re only as smart as the data we pull in. And I think the theme should be fairly obvious by now: that data is king,” Sexsmith said.
If you want to attend sessions like this one at future virtual summits, pre-register today!
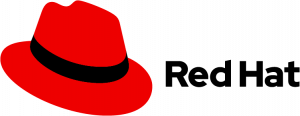
Isaac, great job tackling this challenging topic! AI is very exciting, but I can see how it could get out of hand without the necessary tools (like the Red Hat open data hub)!