The memory of the Office of Personnel Management (OPM) data breach still haunts government. Four years after OPM announced that two attacks had stolen the personal information of 21.5 million individuals – mostly federal employees – agencies are still searching for the best way to prevent breaches like those in 2015, which were not recognized until months later.
In 2019, many agencies are looking to artificial intelligence (AI) and machine learning (ML) as potential solutions, which is why GovLoop hosted a roundtable discussion Wednesday morning to examine how AI and ML can stifle cyberattacks.
“We need to get better at responding to incidents and then heading off attacks,” Pamela Isom, Deputy Chief Information Officer (CIO) and Advisor to the Chief Data Officer (CDO) at the Energy Department (DOE).
AI and ML can rapidly detect gaps or abnormalities on agency networks, and respond with a programmed, precautionary or reactionary action immediately. AI and ML capabilities can patch, contain and prevent network threats.
One example where AI has been deployed to great effect is monitoring for insider threats. If a user profile performs irregular action and acts anomalously, AI can shut down actions from that profile until security has a chance to review the case and contact the person. This can stop willing or negligent insider threats.
AI can also address aging systems by diagnosing important risk factors.
However, AI isn’t a panacea for all of government cybersecurity. In fact, an ill-fitting or hastily enacted solution can encumber business processes or fail to answer existing challenges.
Addressing questions from government attendees, Isom and experts from Cloudera laid out key steps and considerations for how AI could be used to defeat cyberattacks. Henry Sowell, CIO and Technical Director of Solutions Engineering, and Shaun Bierweiler, Vice President and General Manager of Public Sector, from Cloudera offered insight from their experience working with government partners to implement AI and bolster cybersecurity.
The following steps are insights from the panelists and attendees at the event and, while every agency is unique, offer a general framework for an AI journey meant to defend data and elevate agency missions.
- Note: Only the final steps involve AI. The groundwork of data and policy must be laid down first on a successful AI journey.
Five Steps to Beating Cyberthreats With AI
- Establish data standards and governance
Agencies have no shortage of data. In fact, some agencies are overflowing with data, as they have to delete some data assets to make room for others.
Yet for the abundance of data that agencies contain, data quality is sorely lacking across the federal enterprise. Isom pointed out that much of government data is dark data – or information that is unknown. Meanwhile, data that could be shared between networks often lacks standardization and can then present a risk of ownership, as several in attendance from health care agencies added.
Bierweiler emphasized that agencies should designate data responsibilities and roles throughout enterprises. A data strategy, he said, should be created for every agency.
- Modernize IT accordingly
Legacy IT is a given in government, but aging systems alone aren’t the reasons for government’s gridlock of data. Data fails to integrate when systems and policies create silos throughout an organization, and the wrong IT modernization project could lead to the same dilemma if data is an afterthought.
Standard checklists and blanket mandates doom data journeys when agencies fail to consider their users’ unique needs. Bierweiler stressed that while “modernization is absolutely necessary,” a poorly carried out cloud or AI transition might not tear down silos at all, and it could confuse employees.
But by putting data first, modernization can be additive.
“Data is a currency. It is the livelihood of an organization,” Bierweiler said.
Modernization transitions should therefore be centered around data, collaboration and users, and they should never undertaken just because a technology is popular. Instead, target investments that can fit into IT architecture and security structures, improving data mobility and capabilities piece by piece.
- Preserve, improve and create valuable data
Not all data is created equal. Old data that is unstructured or requires manual intervention is significantly less useful than structured, standardized data that technologies can leverage. In fact, poor data quality can lead to inaccurate findings and exhaust resources. Moreover, data without context can easily be disregarded and fall back into the category of dark data.
Data needs to be standardized, aggregated, structured and contextualized so that analytics formulas can actually yield insights. Legacy technologies, for example, that discard data after three months will fail to show trend patterns. And duplicative data that is not cleaned can lead to inconsistent or incomplete results.
Sowell said that first, agencies must capture and retain their data. Then, the correct metadata must be attached to improve data quality. Scoring data, adding enrichments and profiling trends all lead to easier, more useful analytics.
- Develop formulas and datasets to train AI/ML models
It’s almost time to incorporate AI and ML – but not just yet. Central data repositories are necessary to aggregate data from across the enterprise, and agencies need to ensure standardization so that models can be trained.
From this point, AI and ML formulas need to be written, including rules and instructions given the different possibilities of the program. AI and ML are developed with datasets to base their decisions off of and can simulate human intelligence by considering instructions, their inputs and prior decisions. Testing AI and ML with large datasets will help to eliminate anomalies and unpredictable or biased decisions.
- Launch an AI/ML pilot program with users as a focus
“Start small,” Isom said to the room.
With AI and ML, there are consequences of going too big, too fast. Incorrect decisions or overreliance on technology could adversely affect the agency. Instead, start with a high-potential but low-risk pilot program before applying the technology enterprisewide. Early on in the process, agencies can get a sense of the advantages and disadvantages of AI and ML, while educating users on how best to work alongside the technologies.
“AI and machine learning often sound magical. And the whole thing about it is we want it to sound boring,” Sowell said.
Working with users to understand exactly what AI and ML will do is crucial. Disproving notions that it’s taking jobs or going to perform thoughtful decisions, agencies can ensure that the workforce is ready for the technology. Realistically, AI will aggregate, process and deliver the information that users then have to act upon.
Finally, AI isn’t a blanket term. Sometimes, it will shut down user profiles in the name of cybersecurity, following rules established by coders. But other times, it’s just about cleaning data. Agencies just need to be clear what their intentions are in incorporating the technology.
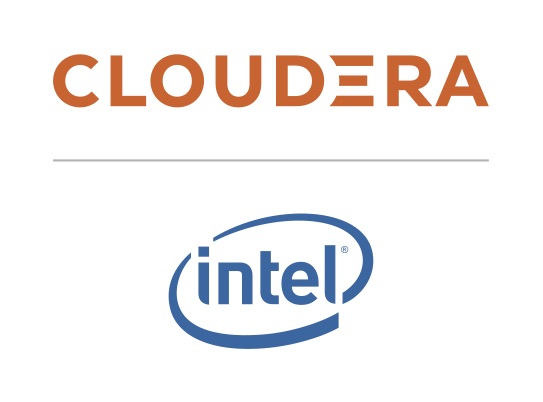
Leave a Reply
You must be logged in to post a comment.